Artificial intelligence (AI) has become more prevalent in a number of various domains, most notably the pharmaceutical industry.
In this review, we focus on how AI is being used in a variety of pharmaceutical industry fields, such as drug discovery and development, drug repurposing, increasing pharmaceutical productivity, and clinical trials, among others.
This use of AI lessens the workload of human workers while also achieving goals quickly. We also talk about how various AI tools and methodologies interact, current problems and solutions, and the potential applications of AI in the pharmaceutical sector.
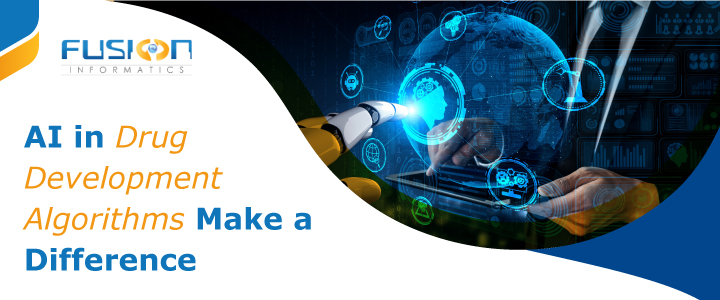
The Fundamentals of AI in Drug Development
AI encompasses a number of approach fields, including machine learning as its core paradigm as well as reasoning, knowledge representation, and solution search (ML). In machine learning (ML), algorithms are used to find patterns in a set of data that has been further categorized.
Deep learning (DL), is a branch of machine learning that uses artificial neural networks (ANNs). These are a group of intricately connected computing components called “perceptons” that resemble biological human neurons in their ability to transmit electrical impulses.
ANNs are made up of a collection of nodes that each process a distinct input before combining them into outputs that may be used singularly or in a network to solve issues. Recurrent neural networks (RNNs), convolutional neural networks (CNNs), and multilayer perceptron (MLP) networks are a few examples of the several types of ANNs that are used in either supervised or unsupervised training procedures.
The MLP network can be used for a variety of purposes, such as pattern recognition, optimization aids, process identification, and controls. It is often taught using supervised training processes that operate in a single direction only.
RNNs, which include Hopfield networks and Boltzmann constants, are closed-loop networks with the ability to memorize and store information. CNNs are a group of dynamic systems with local connections that are distinguished by their topology and are used for a variety of tasks, including the processing of images and videos, the modeling of biological systems, the analysis of complex brain functions, pattern recognition, and sophisticated signal processing.
Among the more complicated types are ADALINE networks, RBF networks, LVQ networks, Kohonen networks, and LVQ networks.
Given that AI can help with rational drug design, support decision-making, identify the best course of treatment for a patient, including personalized medicines, manage the clinical data generated, and use it for future drug development, it is reasonable to assume that it will play a role in the development of pharmaceutical products from the bench to the bedside.
Marketing executives can allocate resources for maximum market share gain, reverse poor sales, and anticipate where to make investments with the aid of AI platforms that use ML algorithms and an intuitive user interface to create analytical roadmaps based on competitors, key stakeholders, and currently held market share.
molecules make up the enormous chemical space, which encourages the creation of many different pharmacological compounds. However, the drug development process is constrained by a lack of cutting-edge technologies, making it a time-consuming and expensive endeavor that can be resolved by applying AI.
It can distinguish between hit and lead compounds, validate the drug target more quickly, and optimize the design of the medicine.
The research and drug development process can take over a decade and cost an average of US$2.8 billion. Even then, nine out of ten pharmaceutical compounds are not able to complete Phase II clinical trials and receive regulatory approval. Algorithms like Nearest-Neighbor classifiers (RF), extreme learning machines (SVMs), and deep neural networks (DNNs) are used for VS and may also predict in vivo activity and toxicity based on synthesis feasibility.
In collaboration with IT companies, a number of biopharmaceutical companies, including Bayer, Roche, and Pfizer, have developed a platform for the identification of medicines for ailments like immuno-oncology and cardiovascular ailments.
Several AI-based techniques can be used to predict physicochemical properties. For instance, machine learning (ML) trains the software utilizing enormous data sets produced during earlier compound optimization.
Drug design algorithms use molecular descriptors to create viable molecules using DNN and then predict their properties. These molecular descriptors include SMILES strings, potential energy measurements, electron density around the molecule, and coordinates of atoms in 3D.
AI drug development companies are currently finding over 150 small-molecule medications, and more than 15 of these are already in clinical trials. It would be unable to accomplish this great achievement in the absence of intelligent systems.
As part of artificial intelligence (AI) in the drug development process, automation is employed in the research and development of innovative medications. It involves using technology to foresee how new treatments will behave in the body and to understand how they work. This speeds up the creation of new medications and improves forecast accuracy.
Data processing capabilities also assist organizations using data science and AI in making such waves in the pharmaceutical industry. Machine learning algorithms are capable of quickly learning from enormous amounts of data and making extremely accurate predictions.
It means that computers are now able to uncover promising therapeutic possibilities by sifting through an endless stream of data.
Benefits of AI drug development
The pharmaceutical sector has faced difficulties as a result of a data overabundance. The industry is unavoidably facing challenges as it progressively transitions to digital. Data processing, inspection, and analysis are important but difficult activities for drug researchers.
The following is a list of the main benefits of artificial intelligence for healthcare in general, with a focus on drug development.
Speed of Analysis time
A brand-new anticancer drug is currently undergoing a clinical trial, which was launched by the German biotechnology company Evotec in 2021. The candidate discovery procedure took 8 months, despite the fact that it seemed routine. Systems with artificial intelligence were directly responsible for these previously unheard-of speeds.
The many characteristics of millions of possible tiny molecules may be automatically compared thanks to advanced technologies. The development and analysis timeframes are shortened since it appears that a significant piece of the analysis process is managed by AI.
Savings
$2.168 billion is the average cost per medicine during the R&D process. The drug discovery phase is where the biggest portion of this money is going. Up to $26 billion in annual resources could be saved on medication screening and synthesizing thanks to AI-based drug development.
Avant-garde algorithms for drug discovery
In order to incorporate the most recent technology into the algorithms, machine learning solution suppliers typically keep on the cutting edge of innovation. AI for drug development offers a distinctive combination of health data and technology when combined with powerful computing capacity. The benefits described above are further enhanced by this combinations
Improved Success Rate
90% of medication candidates drop out of clinical trials during the first phase, according to statistics. This percentage will appear even more intimidating if the failure rate during the preclinical stages is taken into account. By evaluating biomarkers that indicate the efficacy of the treatment, intelligent algorithms can reverse the figures.
Big data for healthcare and artificial intelligence also create a single data layer. Advanced machine-learning algorithms can evaluate a wide range of insights, which helps to classify diseases, with the use of vast volumes of medical data.
When all the information is combined, researchers will have a clearer understanding of the condition and viable therapeutic possibilities for each unique situation.
Summary
The process of developing new drugs is challenging. A medicine must undergo extensive development before it is ready for commercialization, which typically takes a further eight years after FDA approval.
However, AI has the potential to significantly shorten this period while lowering costs and increasing the precision of drug discovery.
Additionally, researchers can avoid developing medications that are harmful or have unintended consequences on people by using AI tools to forecast how molecules will interact.
Despite current challenges, AI-developed drugs are gradually entering the pharmaceutical market. The untapped potential of AI will probably start to take off in the next years as a result of the explosion in data.